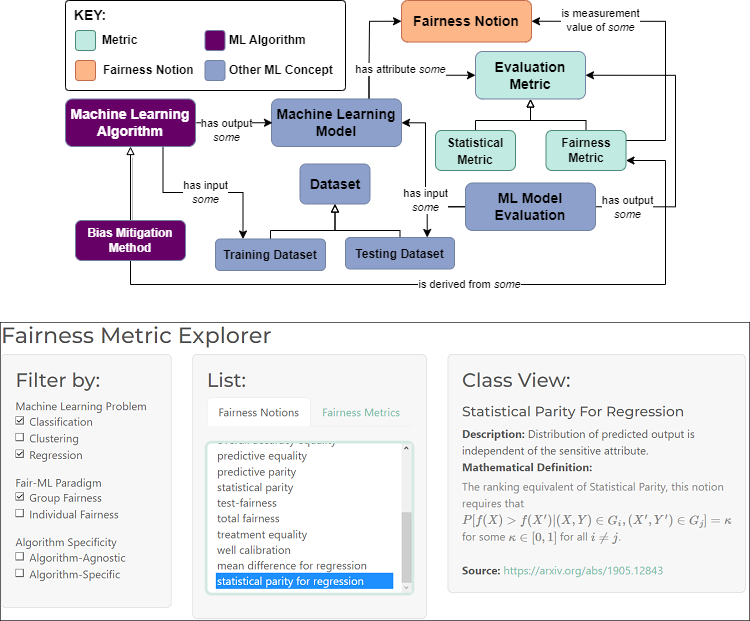
FMO is an ontology for defining and classifying fairness metrics for ML models. It identifies the conceptual notions of fairness that each metric measures, and is intended to guide both ML developers and fairness researchers in understanding the different approaches towards fairness in ML.
For easy access to FMO, we have created the Fairness Metric Explorer web app. The app allows users to search for metrics and notions by selected criteria, and provides a portal to view information about each class in the ontology.
Getting Started: github.com/frankj-rpi/fairness-metrics-ontology
Ontology PURL: https://purl.org/twc/fmo
Fairness Metric Explorer: inciteprojects.idea.rpi.edu/fairness/app/fairness/
- Caton, Simon, and Christian Haas. "Fairness in machine learning: A survey." ACM Computing Surveys. 2023.
- Rismani, Shalaleh, et al. "From plane crashes to algorithmic harm: applicability of safety engineering frameworks for responsible ML." Proceedings of the 2023 CHI Conference on Human Factors in Computing Systems. 2023.
For more citations, see Google Scholar.
Franklin, J. S., Bhanot, K., Ghalwash, M., Bennett, K. P., McCusker, J., & McGuinness, D. L. (2022, July). An Ontology for Fairness Metrics. In Proceedings of the 2022 AAAI/ACM Conference on AI, Ethics, and Society (pp. 265-275). https://doi.org/10.1007/978-3-031-47745-4_18
- Franklin, J. S., Powers, H., Erickson, J. S., McCusker, J., McGuinness, D. L., & Bennett, K. P. (2023, October). An Ontology for Reasoning About Fairness in Regression and Machine Learning. In Iberoamerican Knowledge Graphs and Semantic Web Conference (pp. 243-261). Cham: Springer Nature Switzerland. https://doi.org/10.1145/3514094.3534137