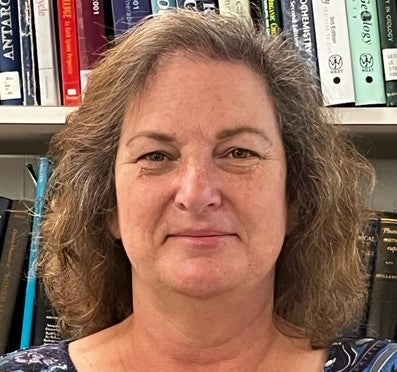
My research interest lies at the intersection of data science, bibliometrics, and prebiotic chemistry. My doctoral thesis focused on leveraging data science methodologies to analyze and understand the evolution of scientific fields, specifically the origins of life and prebiotic chemistry. By utilizing Natural Language Processing (NLP), network analysis, and bibliometric techniques, I have explored the dynamics of scientific collaborations, the historical development of theories, and the intricate networks that connect researchers, institutions, and key concepts within these fields.
My work aimed to construct and validate a comprehensive dataset that captures the key elements of prebiotic chemistry, allowing for deeper analysis of author collaborations, institutional partnerships, and citation networks. This approach provided insights into the structure and progression of scientific knowledge, with a focus on interdisciplinary interactions and thematic trends within the prebiotic chemistry domain.
I am interested in applying these data-driven techniques to further explore scientific disciplines, identify emerging research themes, and uncover hidden patterns of collaboration. My goal is to contribute to the understanding of scientific knowledge creation and dissemination, ultimately supporting the advancement of research in prebiotic chemistry and related fields.