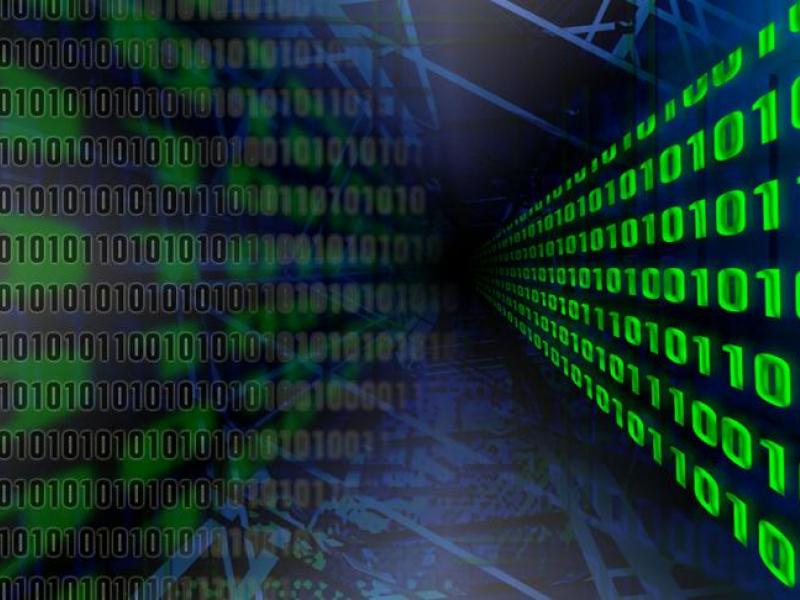
To meet its potential for driving discovery and knowledge acquisition, data science must address the key challenges posed by “Big Data,” assert Rensselaer Polytechnic Institute Professors James Hendler and Peter Fox in a commentary appearing in the June edition of the journal Big Data. Those challenges are not typically represented in university data science programs, which currently emphasize statistical techniques, visualization, and programming/analysis applications.
"Despite all the talk about the need for data science, there has not been a consensus as to what are the real challenges in data-enhanced science and engineering,” said Hendler, director of the Rensselaer Institute for Data Exploration and Applications (IDEA) and the Tetherless World Research Constellation professor of computer, Web, and cognitive sciences. “The Rensselaer IDEA is not just about Big Data, it’s about really understanding the way data is creating new research paradigms across the campus.”
In the commentary “The Science of Data Science,” Hendler and Fox, Tetherless World Research Constellation Chair and a professor of earth and environmental sciences and computer science at Rensselaer, and a member of IDEA, write that “a research agenda is needed that explores the key challenges that must be met to fulfill the needs of research driven by large-scale data analytics.”
First among the challenges are those posed by the “big” in Big Data. Extremely large data sets are difficult to manipulate, and may describe processes on multiple scales – the authors offer an example of a study in which researchers seek to understand interactions between traffic patterns and air pollution, a scenario that will include data from the scale of city roadways to the molecular.
Second, data may be gathered at different sampling rates, complicating attempts to derive mathematical relationships between different processes. And while many systems may be continuous, data may be sampled in discrete units – creating problems related to sparse or inadequate data. These problems are particularly acute in interdisciplinary research, which combines multiple methodologies.
Third, models that rely on data may be compromised by inaccurate measurements, data sampling problems, and cognitive biases. The authors propose that new models of abductive reasoning are needed to automatically generate and test hypotheses for such flaws.
Fourth, the greatest return on data will only come with better and smarter infrastructure – built around semantic technologies – for storing, searching, accessing, and integrating data resources.
In the commentary Hendler and Fox state:
… The science of data science must go beyond reporting the correlative results of data analytics to developing the predictive and prescriptive causal modes that are the basis of science-driven understanding, engineering, and policy making. … It is not enough to know that a particular system has certain properties, but rather to understand, at a systems level, what causes those properties and how, when possible, to manipulate them.
Big Data, broad data, high performance computing, data analytics, and Web science are creating a significant transformation globally in the way we make connections, make discoveries, make decisions, make products, and, ultimately, make progress. The Rensselaer IDEA is a key component of Rensselaer’s university-wide effort to maximize the capabilities of these tools and technologies for the purpose of expediting scientific discovery and innovation, developing the next generation of these digital enablers, and preparing our students to succeed and lead in this new data-driven world.
IDEA serves as a hub for Rensselaer faculty, staff, and students engaged in data-driven discovery and innovation, empowering researchers with new tools and technologies to access, aggregate, and analyze data from multiple sources and in multiple formats. IDEA connects three of the university’s critical research platforms: the CCI supercomputing center (AMOS and Watson at Rensselaer), the Curtis R. Priem Experimental Media and Performing Arts Center, and the Center for Biotechnology and Interdisciplinary Studies.
Contact
Mary Martialay Rensselaer Polytechnic Institute (518) 276-2146 (518) 951-5650 (mobile) martim12@rpi.edu
Visit the Rensselaer research and discovery blog: http://approach.rpi.edu
Follow us on Twitter: www.twitter.com/RPInews