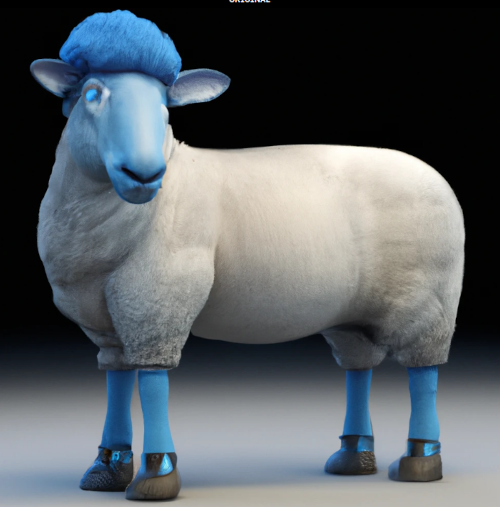
Amos Eaton 214
WHAT: "The Need for Multifactor Bias Benchmarking of LLMs "
LEADER: Hannah Powers
VIDEO: https://youtu.be/kJqnb_hlzo8
EVENT PAGE: https://bit.ly/foci_llm_users_21feb2024
WHEN: 6p, 21 Feb
CONTACT: Aaron Green <greena12@rpi.edu>
DESCRIPTION: LLMs have shown a capacity for producing toxic and biased responses to even innocuous prompts. Bias benchmarks exist to evaluate models for trustworthiness and identify at-risk subgroups from model responses. However, these benchmarks exhibit gaps that bias the analysis. Furthermore, existing analyses lack the statistical foundations needed to make definitive conclusions about the model's biases. We propose a method of identifying gaps in existing benchmarks and a multi-factor bias analysis of LLMs to identify key factors behind model behavior.
BIO: Hannah Powers is a second year PhD student in the computer science department at RPI. Her current research involves evaluating the trustworthiness of large language models. She has an interest in ethical machine learning and artificial intelligence.
Recordings of previous FOCI GenAI Users Group sessions:
- 20 Sep 2023: Introduction to Large Language Models
- 18 Oct 2023: A Guide into Open-Source Large Language Models and Fine-Tuning Techniques
- 15 Nov 2023: "Beyond Autocomplete: Instruction Following & CoT Reasoning in LLM Agents"
- 31 Jan 2024: "The Large Language Model for Mixed Reality (LLMR)"
- 21 Feb 2024: "The Need for Multifactor Bias Benchmarking of LLMs"